Proceedings of the 5th International Conference
on Statistics: Theory and Applications (ICSTA 2023)
August 3 - 5, 2023 | Brunel University, London, United Kingdom
The keynote information for the International 5th International Conference on Statistics: Theory and Applications (ICSTA 2023) is as follows:
Plenary Speaker
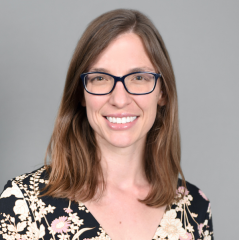
Dr. Melissa Wilson
Arizona State University, USA
Keynote Speakers
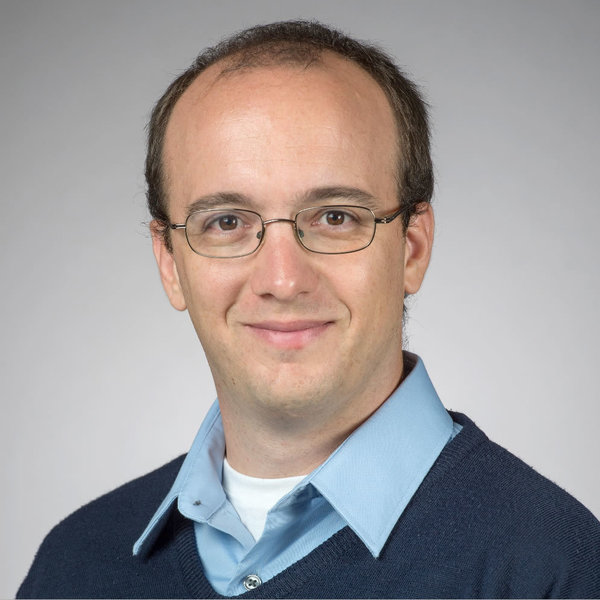
Dr. Stefano Castruccio
University of Notre Dame, USA
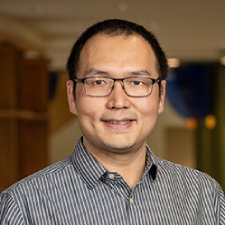
Dr. Jian Kang
University of Michigan, USA
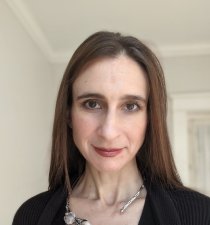
Dr. Cynthia Rudin
Duke University, USA
ICSTA 2023 Plenary & Keynote Speakers
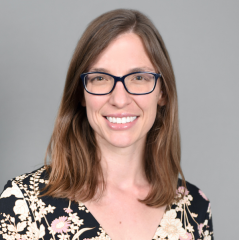
Dr. Melissa Wilson
Arizona State University, USA
Melissa Wilson is a computational evolutionary biologist whose main research interests include sex-biased biology. Her lab studies the evolution of sex chromosomes (X and Y in mammals), how changes in population history affect the sex chromosomes differently than the non-sex chromosomes, and develops novel approaches to incorporating sex as a biological variable into genomics research. Usually she studies mammals, with a particular focus on how the evolution of the placenta shaped sex differences in human health and how sex differences in the placenta may underlie sex differences in the developmental origins of disease. Dr. Wilson completed a Bachelor’s in Mathematics at Creighton University and a Ph.D. in Integrative Biosciences: Bioinformatics and Genomics at Penn State University. She was a Miller Fellow at UC Berkeley before starting The Sex Chromosome Lab at Arizona State University. Although the lab primarily focuses on mammals, being located in the Sonoran desert precipitated additional projects including the study of sex chromosomes in the Gila monster, and population genetics in desert tortoises.
Topic of Plenary:
Sex-Biased Genome Evolution
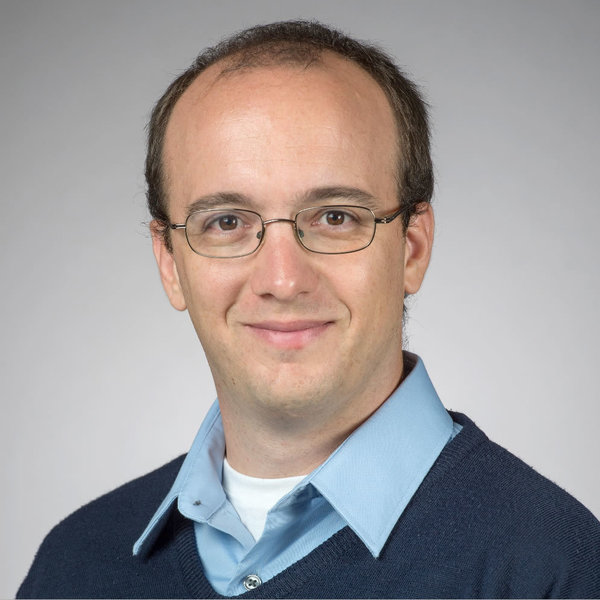
Dr. Stefano Castruccio
University of Notre Dame, USA
Prof. Castruccio obtained his PhD at the University of Chicago (USA), and has worked in Saudi Arabia and the United Kingdom before his current appointment as Associate Professor at the University of Notre Dame (USA). His main area of research focuses on the development of spatio-temporal statistical models for environmental applications, with applications spanning from assessment of renewable energy resources to assessing mortality from air pollution. His focus is mostly on climate and weather modes, and the use of statistical and machine learning models that can act as a stochastic approximation to provide a computationally affordable assessment of parameter sensitivity analysis. His methodological research is focused on the development of non-stationary Gaussian processes in Euclidean and spherical domains, and in scalable multi-resolution inference for high-dimensional processes in space and time.
Topic of keynote:
Stochastic Environmental Modeling in a Time of Convergence: Physics Meets Artificial Intelligence
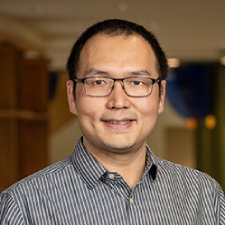
Dr. Jian Kang
University of Michigan, USA
Dr. Jian Kang is a Professor in the Department of Biostatistics at the University of Michigan. He received his PhD in Biostatistics from the University of Michigan in 2011. He was an Assistant Professor in the Department of Biostatistics and Bioinformatics and the Department of Radiology and Imaging Sciences at Emory University from 2011- 2015. His main research interests are developing statistical methods and theory for large-scale complex biomedical data analysis with focuses on Bayesian approaches, imaging statistics and bioinformatics. He has co-authored over 110 publications in statistical journals and medical journals. Dr. Kang currently serves as the Associate Editor of Annals of Applied Statistics, Biometrics, The New England Journal of Statistics in Data Science and Statistics in Medicine. Dr. Kang was elected as the Fellow of the American Statistical Association in 2021.
Topic of Keynote:
Deep Kernel Learning based Gaussian Processes for Bayesian Image Regression Analysis
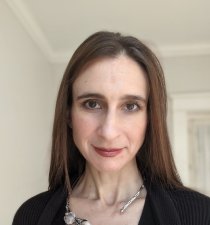
Dr. Cynthia Rudin
Duke University, USA
Cynthia Rudin is Earl D. McLean, Jr. Professor of Computer Science, Electrical and Computer Engineering, Statistical Science, Mathematics, Biostatistics & Bioinformatics at Duke University. She directs the Interpretable Machine Learning Lab, whose goal is to design predictive models that people can understand. Her lab applies machine learning in many areas, such as healthcare, criminal justice, and energy reliability.
Prof. Rudin holds an undergraduate degree from the University at Buffalo and a PhD from Princeton University. She is the recipient of the 2022 Squirrel AI Award for Artificial Intelligence for the Benefit of Humanity from the Association for the Advancement of Artificial Intelligence (the “Nobel Prize of AI”). She is also a three-time winner of the INFORMS Innovative Applications in Analytics Award, and a 2022 Guggenheim Fellow. She is a fellow of the American Statistical Association, the Institute of Mathematical Statistics, and the Association for the Advancement of Artificial Intelligence. She has recently joined the US National AI Advisory Committee (NAIAC) as a member of the Subcommittee on AI and Law Enforcement.
Topic of Keynote:
Understanding How Dimension Reduction Tools Work